Welcome to Just Two Things, which I try to publish daily, five days a week. Some links may also appear on my blog from time to time. Links to the main articles are in cross-heads as well as the story.
#1: Playing outside
Children are losing the habit of playing outside. This was true before the pandemic, but the experience of lockdown has made it worse.
(Image from Classroom Secrets)
Over at The Conversation, two academics argue that getting back into the habit of playing outside—and even better, unsupervised—should be a priority once the pandemic is over.
The argument goes like this.
There’s a huge body of research evidence now that shows that active outdoor play has benefits right across the spectrum of child development—for physical and psychological health, for wellbeing, for development and for educational attainment. Free play, which is unsupervised and not organised by parents or teachers, is especially good for wellbeing, development and resilience.
But one of the costs of lockdown has been that children have spent more time indoors, have become less physically active, and have spent more time in front of screens. In some places children have been reprimanded by police for playing outdoors. In England this may be the least fit generation in history, although measurements are poor (which is another problem).
Parents give lots of reasons for not having kids play outside:
fear of children hurting themselves or getting dirty, stranger-danger, sunburn, insect stings, bad weather and darkness. These safety concerns are conveniently countered with lots of “safe” things for children to do indoors, mostly in front of screens.
The problem isn’t access to outdoor spaces. A study in Scotland found that most children had access to decent outdoor play spaces that were considered safe. The same is true across the rich world. Meanwhile, the indoor environment is less safe than parents think, according to Canadian research.
The researchers don’t have any specific recommendations, beyond suggesting that families need to get out more, to build up an active play habit. That sounds like a recipe for improving outcomes for the better off, not for everybody. And this also sounds to me like one of those classic public policy problems where a focus on immediate risks blinds us to the wider long-term issues. It’s similar to cycling, where the risk of being hit by a car is visible and immediate, but the larger long term public health gains from cycling aren’t seen. It will probably need a proper policy intervention.
#2: The ethics of AI
A couple of weeks ago I mentioned here that Google’s removal of its leading AI ethics researchers over an academic paper on which they were co-authors had turned a welcome spotlight on the subject. The paper has now been published, and John Naughton discussed it in his Observer column last Sunday:
It provides a useful critical review of machine-learning language models (LMs) like GPT-3, which are trained on enormous amounts of text and are capable of producing plausible-looking prose. The amount of computation (and associated carbon emissions) involved in their construction has ballooned to insane levels, and so at some point it’s sensible to ask the question that is never asked in the tech industry: how much is enough?
The paper itself is academic, reasonably concise, and not too technical, at least once you get through the acronyms at the front. The conclusion summarises the argument in this way:
We have identified a wide variety of costs and risks associated with the rush for ever larger LMs, including: environmental costs (borne typically by those not benefiting from the resulting technology); financial costs, which in turn erect barriers to entry, limiting who can contribute to this research area and which languages can benefit from the most advanced techniques; opportunity cost, as researchers pour effort away from directions requiring less resources; and the risk of substantial harms, including stereotyping, denigration, increases in extremist ideology, and wrongful arrest.
The ethics issues of AIs and their related machine learning sets have been reasonably well covered elsewhere. We know that they replicate the bias of the language sets they learn on, and that these language sets are more likely to reflect the speech of those who are already privileged, and de-privilege the already marginalised. The language models “run the risk of ‘value-lock’, where the LM-reliant technology reifies older, less-inclusive understandings.” There is a detailed discussion of the multiple layers involved in this process in the paper, and a memorable quote:
We recall again Birhane and Prabhu’s words (inspired by Ruha Benjamin): “Feeding AI systems on the world’s beauty, ugliness, and cruelty, but expecting it to reflect only the beauty is a fantasy.”
The immense scale is also an issue. The paper notes the risk of “documentation debt”, where sheer size means that the datasets that sit behind the machine learning are both undocumented and also too large to be documented afterwards:
While documentation allows for potential accountability, undocumented training perpetuates harm without recourse. Without documentation, one cannot try to understand training data characteristics in order to mitigate some of these a test of issues, or even unknown ones.
The cost of a big training set has been less well covered. A couple of weeks ago Exponential View linked to a trade industry article that said that in five years time a large language set could well cost $1 billion to train. If that seems a lot, the sums suggest that the hardware alone to drive one of these big language models could easily be in the $150-170 million dollar range.
And if that still seems high: some researchers who’d been trying separately to model the same costs tweeted that they’d reached a figure of a billion dollars—but then decided that the number seemed far too high, and so they fudged their numbers back down to $100 million. It was honest of them to own up.

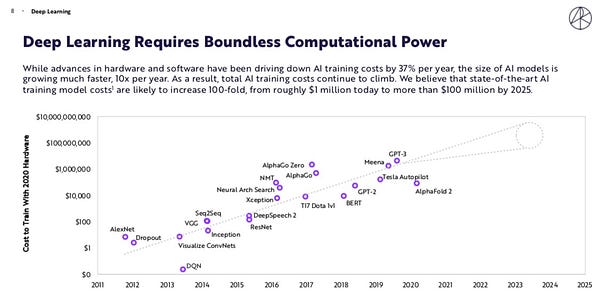
Of course, this price tag suggests to me that only certain people are going to play in this space—and that they will be under strong pressures to monetise their investment, which may mean that ethics vanish pretty quickly.
On environmental impact, The Register reported last year that the ecological impact of training an AI set had increased by a factor of 300,000 between 2012 and 2018. The same researchers estimated the carbon cost of training the GPT-3 AI as being 85 tonnes, or the equivalent of driving a car to the moon and back. Some of the more recent LMs are considerably larger.
(Source: Bender, Gebru et al, 2021, p2)
As Naughton observes, succinctly: “[C]urrent machine-learning systems have ethical issues the way rats have fleas.”
What I took away from the paper, although they don’t say this, is that the public harm from these large language models seems to be spiralling, but with little likelihood of comparable public benefit. And this now does seem to be spilling over into the space of activism. John Naughton, again, notes that there is now
“a student campaign – #recruitmenot – aimed at persuading fellow students from joining tech companies. After all, if they wouldn’t work for a tobacco company or an arms manufacturer, why would they work for Google or Facebook?”
j2t#058
If you are enjoying Just Two Things, please do send it on to a friend or colleague.